Task 1.3 Benchmark Exercise
Verify and Validate the improvements through a common data set to test model results upon and discuss at IEA Task meetings.
- Aim: Collect common data sets for NWP model development, and set up a benchmark process.
- Partners: PNNL, NREL, DWD, DTU, MESO, WEPROG
Building on the verification and validation work developed under the Second Wind Forecast Improvement Project, the work here exhibits the value of a consistent procedure to evaluate wind-power forecasts and represents a contribution to Phase II of Task 36: Wind Power Forecasting of the International Energy Agency’s (IEA’s) Wind Technical Collaboration Programme (TCP).
WE-Validate Code Base
The group has established an open-source Python code base tailored for wind-speed and wind-power forecast validation, WE-Validate. The code base can evaluate model forecasts with observations in a coherent manner. To demonstrate the systematic validation framework of WE-Validate, we designed and hosted a forecast-evaluation benchmark exercise. We invited forecast providers in the industry and academia to participate and submit forecasts for two case studies. We then evaluated the submissions with WE-Validate.
The Code is open source. The code and detailed instructions for users can be found on the GitHub page: github.com/joejoeyjoseph/WE-Validate. The tool is currently tailored for wind-power forecast evaluation, and can be extended to solar forecasting and other applications.
The Benchmark Case
The validation team asked the participants to submit 30-minute forecasts for 2 cases:
- WFIP2 case: Meteorological measurement field campaign targeting the Pacific Northwest of the U.S. The region has complex terrain and onshore wind farms. The measurements were from a SODAR.
- European case: EnBW Baltic 2 Offahore Wind farm and wind data from the FINO 2 met mast platform in the Baltic Sea
For the WFIP2 case, we asked for wind velocity forecasts over 2 days; for the European case, we asked for wind velocity and plant-level power forecasts over 7 days. We requested the participants to submit forecasts aligning with the metadata of the observations, which allowed for valid comparisons between forecasts and observations as well as comparisons among forecasts. We also asked the participants to provide metadata of their numerical models, including the resolutions of the model grid cell and the differences between the ensemble members.
Findings
Our findings suggest that ensemble means have reasonable skills in time-series forecasting, and the code shows correctly that ensemble forecasts need to be applied differently than just using with an ensemble mean, when used for wind-ramp forecasting. Adopting a voting scheme in ramp forecasting that allows ensemble members to detect ramps independently leads to satisfactory skill scores. We also found and want to emphasize the importance of using statistically robust and resistant metrics as well as equitable skill scores in forecast evaluation.
The full report will be published in the Publication Section under Articles & Reports
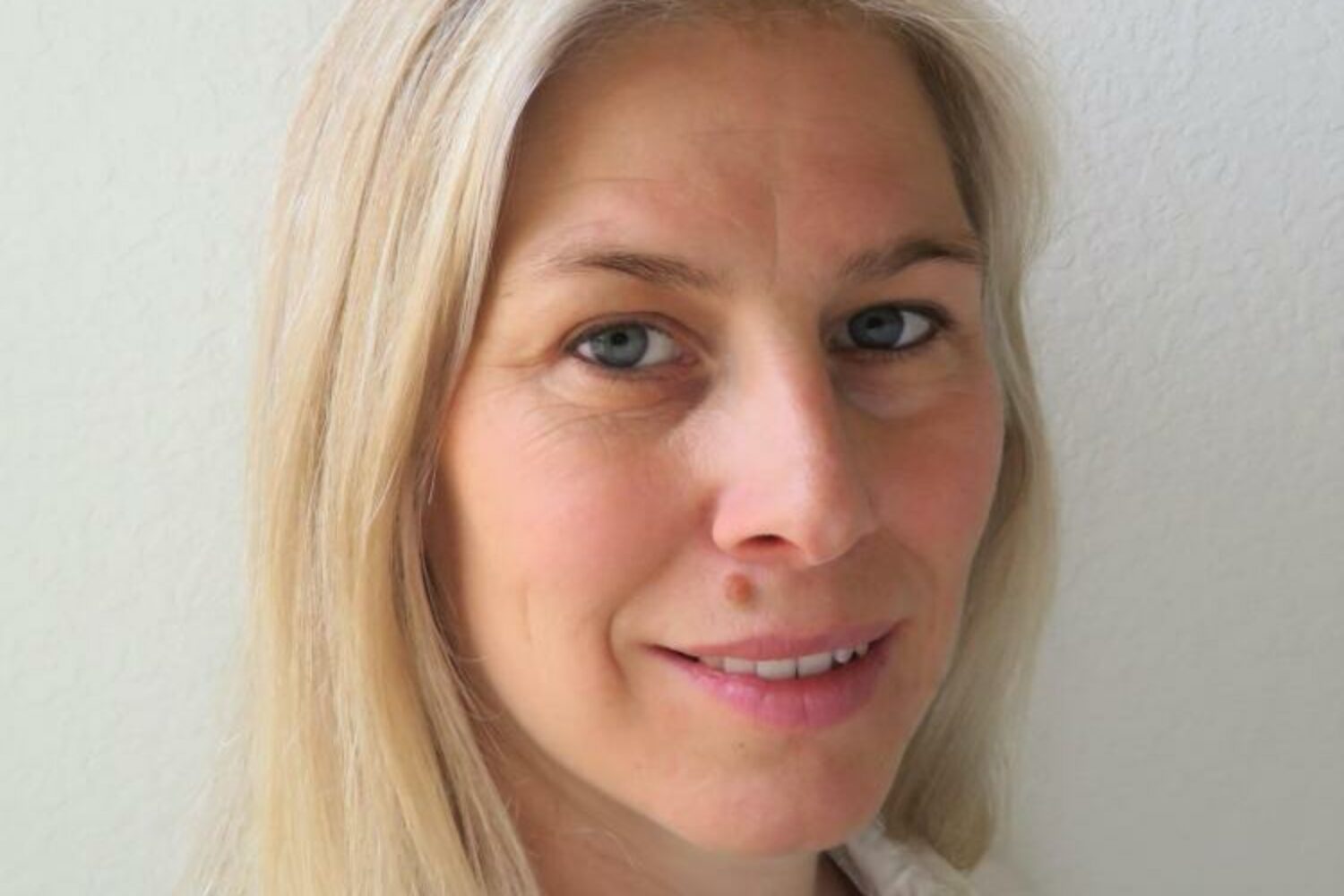
Lead
Caroline Draxl
National Renewable Energy Laboratory
Caroline.Draxl@nrel.gov
Contact: Pacific North-West National Laboratory
Joseph Cheuk Yi Lee
joseph.lee@pnnl.gov